Verifying data quality and evaluating its impact on sales effectiveness
An effective marketing sales strategy today should be based on data, rather than just intuition and general best practices. A data-driven approach to marketing ensures that communication and brand messaging fulfills what’s intended to do: Closes!
However, not every data you have in your database is useful.
Data of low quality such as incomplete details or mismatched fields can mess up your sales processes. Actionable data of good quality enables you to personalize customer experiences and deliver marketing materials that are super-relevant and helpful to your prospects.
But what exactly is data of good quality?
Data of Good Quality
Data quality is a very important phenomenon in the world of data science and data marketing. At a time when marketing is being embraced as both a science and an art, data is increasingly becoming a very important component in realigning the focus of business marketing. They help individuals and brands to make rational business decisions and enable marketing departments to develop marketing content that is tailored to specific target markets.
Characteristics of Data of Good Quality:
The quality of your data relies on 5 major factors including:
- Relevance: Only collect such data that you will actually use for your communications. Asking for a physical address on the checkout page when you clearly don’t need it nor planning to use it in future is just making your checkout process unnecessarily long, increasing the rates of abandoned carts.
- Accuracy / precision: This refers to the exactness of data. Data with inaccurate information can have you sending marketing messages to people who are not the intended recipients.
- Legitimacy and validity: All the entries in a datasets must be valid, based on the field types. Your emails should only accept email addresses; phone numbers, only numbers, and + sign; and universal items such as Gender, nationality, and languages should be limited to a set of options.
- Timeliness: People’s data change over the years and so, data can only be said to be of good quality if it’s up to date. When people stop working for given companies, they usually have their work emails disabled or forwarded to someone else in the company.
- Completeness and Comprehensiveness: Incomplete data is just as dangerous as inaccurate data. You need a complete picture if you are going to use your data to make informed decisions that could see an overhaul in your business systems and processes. Missing data could also mean broken messaging resulting in poor customer experiences and potential exits.
- Consistency: Data has to be the same across all of data sources. This can be hard to achieve, but with integrations available, you can easily update the information about your customers on different platforms automatically. For instance, if you get a new WooCommerce customer, you can set an automation for a new row entry to be created in your Google Sheets. This way, your spreadsheets will always show a true and current state of all the entries of your current customers.
We’ve mentioned that it’s possible to have data of poor quality, but what does that mean in the world of eCommerce?
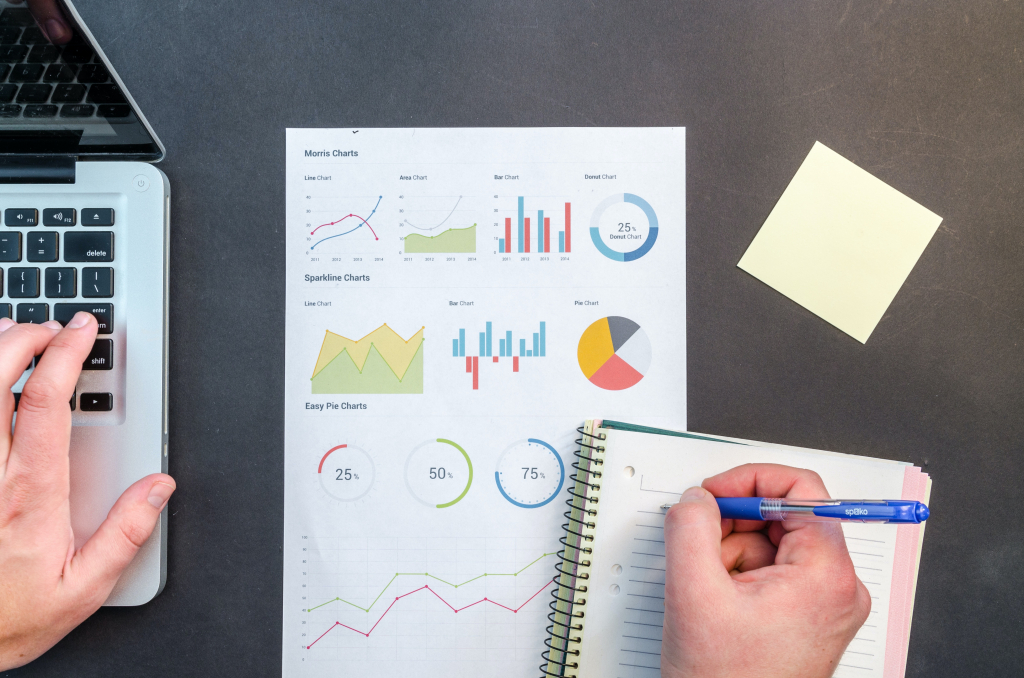
Data of Poor Quality
This is the data that lacks in terms of accuracy, relevancy, validity, completeness, consistency, and timeliness.
This data, when used to make informed business decisions, or for marketing purposes, may harm business and even destroy reputation, both with your customers and ISPs. Bad business decisions often lead to financial losses that can be costly to your business.
Examples of poor-quality data include:
- Duplicate content,
- Mismatched customer data,
- Unstandardized data where different entries enter use different formats. For instance, United Kingdom and UK, $100 and 100$, etc.
- Incomplete data, where major fields are missing from a customer’s data.
What Can Be Potential Causes of Poor Quality Data?
The main causes of poor-quality data include:
Multiple sources of data: if one relies on multiple systems to collect data, then there are chances that one will end up with duplicate content if systems are not synced or integrated properly seem to be much higher.
Lack of guiding standards in entering and handling data: unstandardized data is often a result of people entering the data without following certain guiding formats. For instance, it’s a good practice to always lay out how:
- Names are entered, usually as title case e.g., Katherine Harris, instead of Katherine Harries.
- Job post titles should be entered: (Chief Executive Officer, or CEO).
- Revenues are entered ($10,000 or $10K).
- Numbers are used within your databases.
Data Decay: Recent research by HubSpot has shown that collected data decays at a rate of 22.5% annually. This means that if you don’t take the time to maintain your data accordingly, 22.5% of your database items will be irrelevant. Imagine sending out your marketing campaigns to a list of people knowing that 22.5% of them will not receive them. Think of this as your company annoying 22.5% more people every year! People change email addresses, switch jobs, venture into new fields, get married etc.
Consequences of Using Poor Quality Data
Using data of poor quality in your business or organization can be detrimental in terms of the effects that the consequences can have on your business.
Inaccurate targeting often results in loss of potential revenue: picture this, you want to send a super-relevant marketing campaign to your prospects who average $10K in revenues, but since some of your prospects have entered these data as $10,000, they won’t receive the campaign. If a single order is worth $1000, then you can only imagine how much money you would be losing every time you send a similar campaign.
Poor customer experience often results in the loss of customers: sending irrelevant offers to your customers and prospects due to poor segmentation can lead to annoyance. Annoyed customers are always closer to that unsubscribe button than you think. Also, no one loves receiving promotional campaigns about products they have already purchased from you.
Ineffective marketing that often results in wastage of the marketing budgets and team’s times: inaccurate and old data that is no longer relevant can have your marketing team targeting irrelevant people in a bid to create more targeted ad campaigns. Though a good strategy, no one wants to receive a promotion about growing a company from which they were fired years ago or one that collapsed and went into bankruptcy.
To avoid all these inefficiencies, you need to have a way of enriching and qualifying your data to ensure that they are always accurate and up to date.
Broken Business Processes: Since most business processes are interlinked, a breach in the flow of data at one point of the process affects the entire system. This, therefore, means that the errors are carried all the way to the bottom of the processes, and often when they are covered it’s too late, besides, fixing them can be quite costly. Moreover, some damages are not just repairable.
Damaged Reputation: If you are sending marketing campaigns to dead emails that will never be opened, your open rates within your email service provider will be super low. If people are no longer interested in your content since their life dynamics have changed tremendously over the past two years, they will mark your campaigns as spam. These actions don’t sit well with your Internet service providers (ISPs). Your domain reputation is damaged, and your emails will start getting marked as spam automatically. This can affect your sales and thus handicaps your growth.
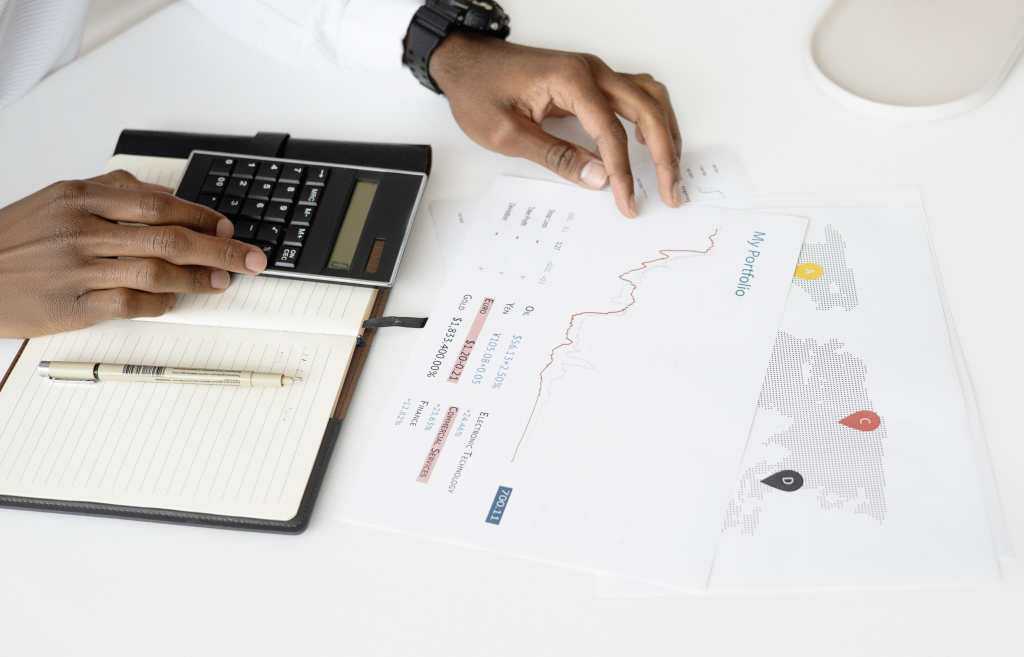
Ways of Qualifying the Quality of Data
To improve the quality of your data, you need to perform 5 main processes:
Data Standardization: Unless you have a laid-out standardization policy in place that is being followed actively, chances are that your data is not standardized. Having different iterations of similar entries is rendering your data ineffective. Standardizing data ensures that entries entered have the same structure. Develop guidelines on how details such as job positions, phone numbers, physical addresses, first names, etc. are to be entered into your database or CRM.
Data de-duplication: This is a process of ensuring that you don’t have different entries of the same data. Some users enter their details in different places, and they may show up in CRM as two different individuals. Duplicate data may not only cost you money but also reduces the effectiveness of data analysis.
Data Normalization: Normalized data makes it easy to analyze and derive conclusions from. It ensures that there are no logical and structural inconsistencies in datasets that may make it difficult to quickly access and retrieve certain information as and when it’s needed.
Data Enrichment: For data to continue being useful, they need to be maintained and improved. Data enrichment is a process of combining the first-party data with disparate data from other internal sources or external sources. Enriched data is more insightful since the entries have more details.
Data validation: The last process is one that involves verification of all the entries in a database to ensure that they are valid. Here, the task is to check for accuracy and inconsistencies, especially after the data have been migrated from one source system to another. Data validation allows irradiating redundancies in data.
There are various data validation and verification tools that can be used to make the process easier. For instance, one can use email verification tools to ensure that all the emails in datasets can deliver campaigns.
Using Ready-made Datasets
Well, it’s apparent that sourcing data and maintaining them is quite an involving and tedious process, which can be quite costly even if you decide to outsource data qualification services.
A work-around to this is using ready-made datasets.
Datasets are sets of information usually around particular industries that have been thoroughly filtered, cleansed, and improved. The datasets are usually provided in ready-to-use formats, either in Excel or CSV file formats.
Verification of quality data which is done on one’s own time is time-consuming, expensive, and often eats into the valuable business hours that are better off spent in activities that improve the bottom line. By using services such as those of Datashop24, you are assured of error-free ready-to-use data.
Our datasets around speed cameras in various countries are now available for download. We have free samples that you can download right away. To access all the entries, simply add to cart the dataset from your country and enjoy our launching pricing.
Dataset | Total Entries | Action |
Speed Cameras in Russia | 746 | Download free samples |
Speed Cameras in the Netherlands | 433 | Download free samples |
Speed Cameras in Great Britain | 1948 | Download free samples |
Speed Cameras in France | 2766 | Download free samples |
Speed Cameras in Switzerland | 462 | Download free samples |
Speed Cameras in Poland | 761 | Download free samples |
Speed Cameras in Italy | 2684 | Download free samples |
Speed Cameras in Germany | 4494 | Download free samples |
Speed Cameras in Austria | 857 | Download free samples |
Explore all the datasets of speed cameras or go to the specific country using the table above.